Three signs that AI predictive analytics fails for your marketing strategy
Are you struggling to adopt AI and predictive analytics in your marketing strategy? The three issues presented in this article are likely what’s holding your organization back.
These days, you can hardly meet a CMO (Chief Marketing Officer) who doesn’t realize that modern marketing must become AI-analytics-driven.
Many businesses have been charging ahead with bold investments in marketing predictive analytics and artificial intelligence (AI). Many marketing executives have dedicated a lot of their own time to implementing AI-enabled analytics campaigns, appointing chief analytics officers, and hiring all sorts of data scientists and engineers.
Here are three red flags signaling that your marketing strategy lacks AI and predictive analytics.
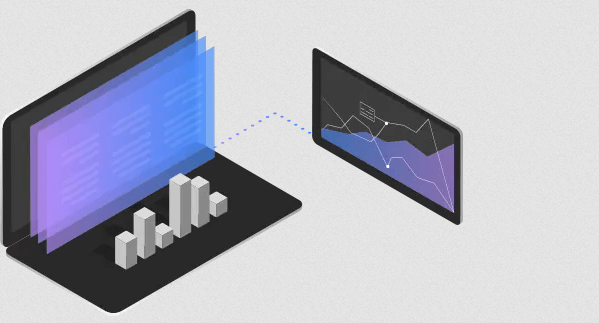
1. Your marketing executive doesn’t realize the power of AI predictive analytics
This situation typically stems from marketing executives lacking a solid understanding of the difference between traditional marketing analytics (that is, marketing intelligence and reporting) and predictive analytics (powered by artificial intelligence and machine learning algorithms).
Here is an example.
One company has invested a lot in data analysts, AI engineers, and other digital roles to build an advanced analytics system for data-driven marketing and lead generation. The CMO regularly mentioned that the firm was using AI technologies, but never with any details.
In fact, the company ran a lot of pilot marketing programs using AI and machine learning techniques, but not a single program was successfully adopted and scaled by the business.
What was the reason?
Senior management didn’t really have a clear vision for the concept of predictive analytics and marketing AI. The CMO struggled to solve pressing problems for the analytics team, and this executive never invested in building the right skills.
That said, the top management lost traction with their marketing AI initiative. The analytics team wasn’t working towards solving the central problem (analyze marketing data and generate accurate predictions) and wasn’t able to get the most of predictive analytics and AI tools.
2. There’s no data analytics strategy in place
The CMO of one eCommerce company was excited about marketing predictive analytics that generates actionable insights from raw data. This executive had several specific cases where she was sure the AI could add value.
However, there was no strategy for how to analyze the data and generate insights beyond those specific cases.
Meanwhile, a competitor began using artificial intelligence to launch a marketing analytics platform that enabled entirely new product and service categories.
By exploiting marketing analytics opportunities in an unstructured way, the competitor achieved more returns and got a chance to capitalize on a bigger opportunity in the future.
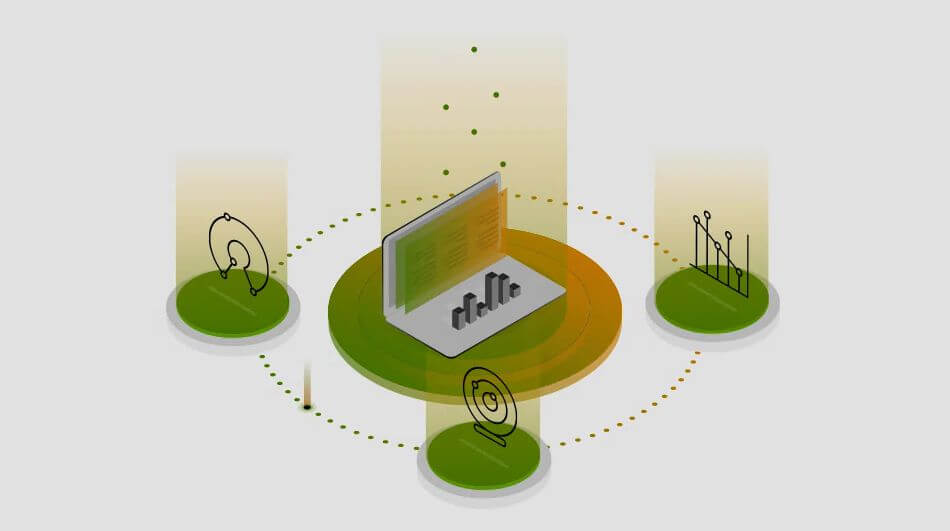
Moreover, it will be easier for the competitor to energize its workforce to imagine what data and insight analytics opportunities lie ahead.
AI-powered marketing predictive analytics requires its own data strategy just like any major business initiative.
Marketing executives should lead an initiative to develop and implement a comprehensive data strategy for all the marketing analytics efforts in 2-3 years ahead.
In this context, an immediate initial step would be to inventory all of the data sources currently being used within the organization.
And then the next step is to encourage buy-in for future analytics investments by scaling analytics use cases and identify 3-5 most data-rich sources that can create the greatest value quickly.
To consider a robust data strategy in the context of marketing analytics AI, think through the following questions:
- Is the data needed of every source accessible and of sufficient quality and time horizon?
- What specific process steps would need to analyze and get actionable insights from a particular source?
- Would the team involved in data collection and analytics need to change and how?
- What would require parallel processes in marketing and beyond until the predictive analytics approach was adopted?
3. The role of data analyst in marketing AI is poorly defined
Today, few marketing executives can really describe in detail what a data analyst does in their organizations and whether or not that employee has the necessary skills, experience and knowledge to take on the role.
Here’s an example.
In one company, the CMO was super-enthusiastic about using AI and predictive analytics in strengthening the marketing mix.
She was especially proud that the marketing team had got 5 data scientists, each at an average cost of $225,000 a year. These people were responsible for collecting and interpreting the data and helping the CMO understand the numbers behind their marketing strategies.
In 6 months, however, after it became apparent that the data analysts were not delivering what was expected, it was discovered that none of these employees were, by strict definition, data scientists at all. Those people could not even explain what marketing predictive analytics means and how AI could help gain a better understanding of which campaigns were working best.
There were no clearly and comprehensively defined responsibilities of the data analyst role, which was the route problem in the organization. Neither the CMO, nore the firm’s HR group had a clear understanding of the data analyst role, and hence the marketing team onboarded incompetent specialists who failed to leverage AI-powered marketing analytics and accurate predictions.
A strategic solution could be that the CMO together with the CTO (Chief Technology Officer) and the HR team would lead an effort to define the role of a marketing data analyst and create detailed job descriptions.
This effort would allow to assess all of those specialists currently with the organization who could meet those job requirements. And then get new hires for the role externally.
No time to waste
There is no time to waste. If you want your digital marketing strategy to succeed you need to adopt predictive analytics and artificial intelligence in your daily tactics. The upside is too significant for it to be discretionary.
But you have to be careful. You don’t want to be caught up in the hype and rush into marketing AI initiatives that cost vast amounts of money and return very little.
Take a look at these three signs presented in this article and find ways to convince your CMO and marketing staff to start adopting AI and predictive analytics.